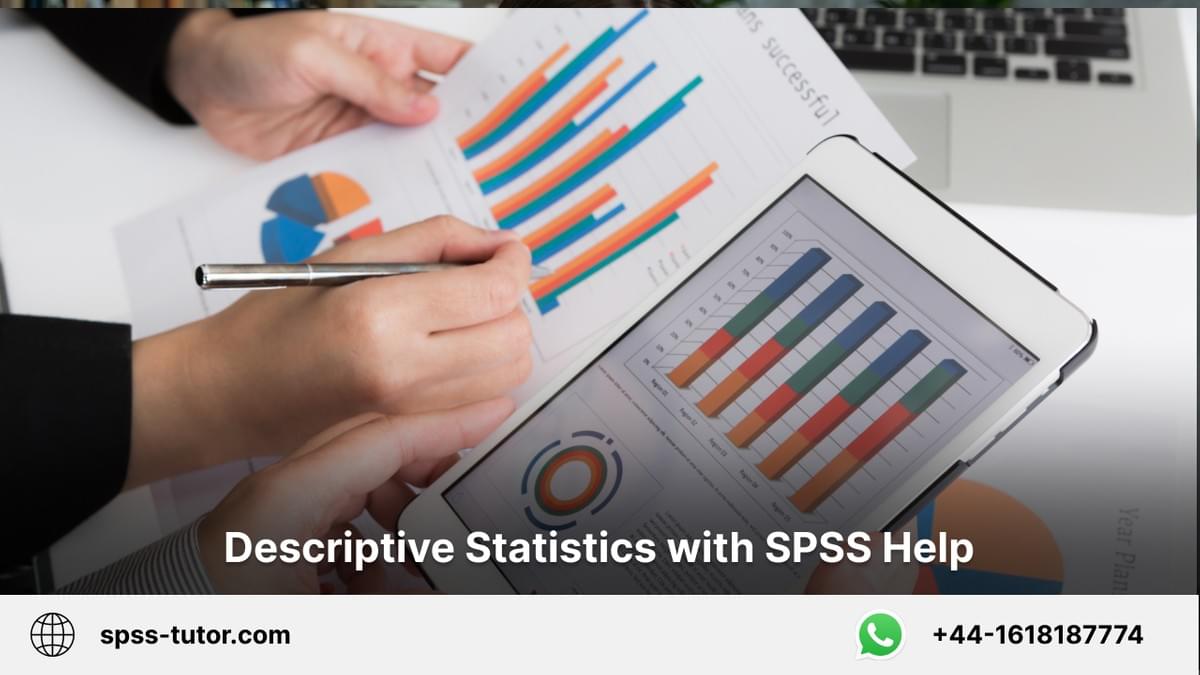
Introduction
Descriptive statistics is the very first step towards data analysis. Researchers who get SPSS help must be aware of this fact. Descriptive statistics help researchers to quantify their data set as well as support them in defining its basic characteristics. Since the process of data analysis begins at this stage of this statistics, researchers can organise, simplify, and summarise their data sets. We are providing this blog to make you understand everything about descriptive analysis, that is its definition, its types, and its examples. Let’s scroll through the blog further.
What Are Descriptive Statistics?
Descriptive statistics provide concise summary measures of a dataset, which can either represent an entire population or a sample from it. They are composed of two types of measures: measures of central tendency and measures of variability (or spread). The mean, median, and mode are examples of measures of central tendency, while measures of variability include standard deviation, variance, minimum and maximum values, kurtosis, and skewness.
Understanding Descriptive Statistics
In brief, descriptive statistics aid in characterising and comprehending the attributes of a particular dataset by providing concise overviews of the sample and its measurements. Measures of central tendency, such as the mean, median, and mode, are the most widely recognised types of descriptive statistics and are utilised across various levels of mathematics and statistics. The mean, which is the average, is determined by summing up all the values in the dataset and dividing by the number of values in the set.
Researchers utilise descriptive statistics by summarising unfathomable quantitative insights into smaller pieces of descriptions for larger data sets. This can be explained using the grades of a student. A GPA operates on the concept of consolidating data points from diverse exams, courses, and grades and computing an average to present a general assessment of a student's overall academic achievement. Essentially, a student's individual GPA represents their average academic performance.
Types of Descriptive Statistics
- Central Tendency
Measures of central tendency determine the intermediate value of the data set and measures of variability determine the dispersion of data. These two central tendencies showcase the analysed data set through graphs, tables, and general discussions so that readers can understand the data easily. Measures of central tendency help identify the epicentre of the distribution of the data set. The mean, median, or mode are usually used when describing the frequency of data points in the distribution, which measures the most common patterns.
- Measures of variability
Measures of variability help in understanding how the data is distributed for a data set. In the case of central tendency, we only get the average value of the data set but the distribution of the values remains unclear. For example, the average of a data set of 100 values is 40 let’s say, but it might be possible that there are values present at 1 and 100. The measures of variability will help us identify them by the shape and spread of the data set. Quartiles, range, variance, and absolute deviation are considered to be examples of measures of variability.
- Distribution
Here distribution refers to the frequency distribution. It allows us to determine the number of times a data point appears. Alternatively, it allows us to determine those data points that are not occurring.
Comparison of Univariate & Bivariate data
Univariate data refers to a single set of data or a variable that is being analysed in isolation. This type of data analysis focuses on describing and analysing the characteristics of a single variable, such as distribution, central tendency, and variability.
For instance, Suppose there is a room filled with people, and you aim to obtain the mean age of the individuals present. In this case, the univariate data pertains solely to a single factor: each person's age. You can compute the average age by collecting this solitary piece of information from each person and dividing the sum by the total number of people.
Bivariate data, on the other hand, involves the analysis of two variables simultaneously. The relationship between the two variables is studied to determine how they are related or correlated. This type of data analysis can provide insights into cause-and-effect relationships, associations, and patterns between the two variables. Bivariate data analysis commonly involves techniques such as correlation analysis, regression analysis, and scatterplots.
For example, In the previous scenario, assume that each person takes a driving test, and the objective is to determine if older people perform better than younger people. To achieve this goal, besides obtaining the peoples' ages, we also need to collect their respective test results. Then, using data analytics, we can represent the relationship between people's age and test scores either mathematically or graphically to observe if any correlations exist between the two variables.
Comparison of Descriptive Statistics & Inferential Statistics
Descriptive statistics and inferential statistics are two different branches of statistics that serve different purposes.
Descriptive statistics refers to techniques used to describe and summarise data. It involves computing various measures such as mean, median, mode, standard deviation, variance, and range, which provide insights into the distribution and characteristics of the data. Descriptive statistics are mainly used to provide a better understanding of the data being analysed.
Inferential statistics, on the other hand, is the process of using a sample to make inferences about a population. This involves analysing data from a sample and using statistical techniques to make predictions about the characteristics of a larger population. Inferential statistics are mainly used to test hypotheses, estimate population parameters, and make generalisations about a population based on a smaller sample.
In summary, descriptive statistics are used to describe and summarise data, while inferential statistics are used to draw conclusions and make predictions about a larger population based on a smaller sample.
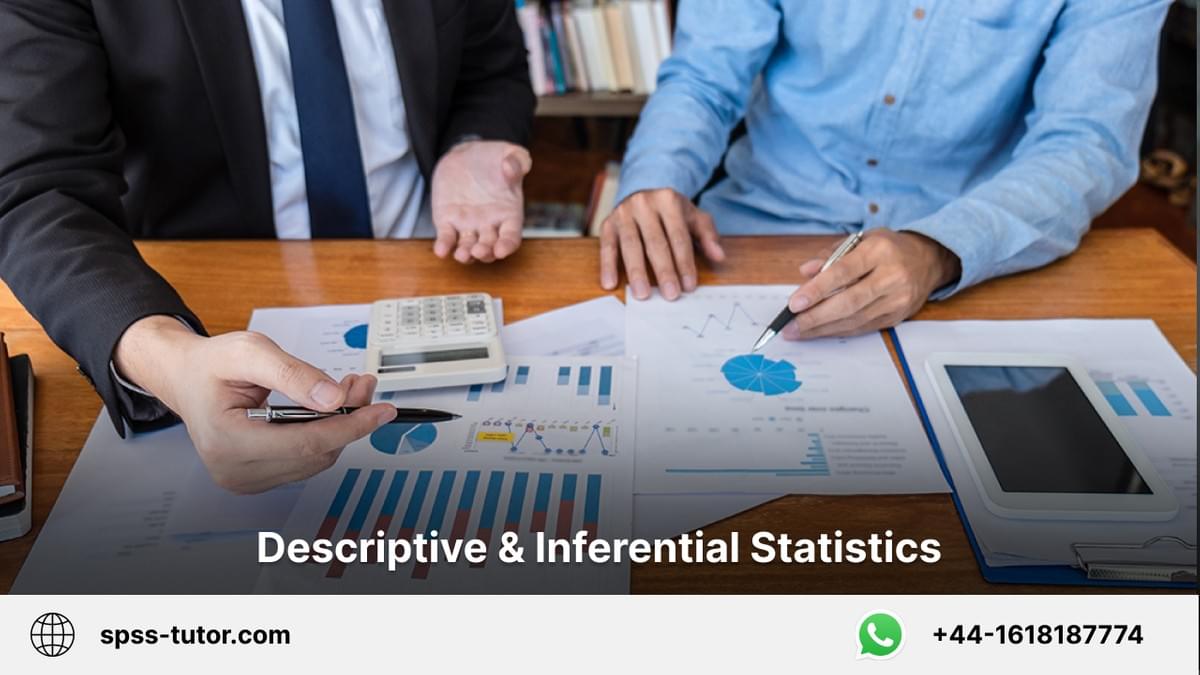
Conclusion
As we have explained, descriptive statistics means analysing and summarising the data set. Although descriptive statistics are rarely useful for decision-making, they can still be useful when summarising high-level information such as mean, median, mode, variance, range, and count. We hope reading this blog has helped you gain a better understanding of descriptive statistics. Otherwise, you can count on the SPSS data analysis services also, which are provided by many online service providers. We wish you good luck!