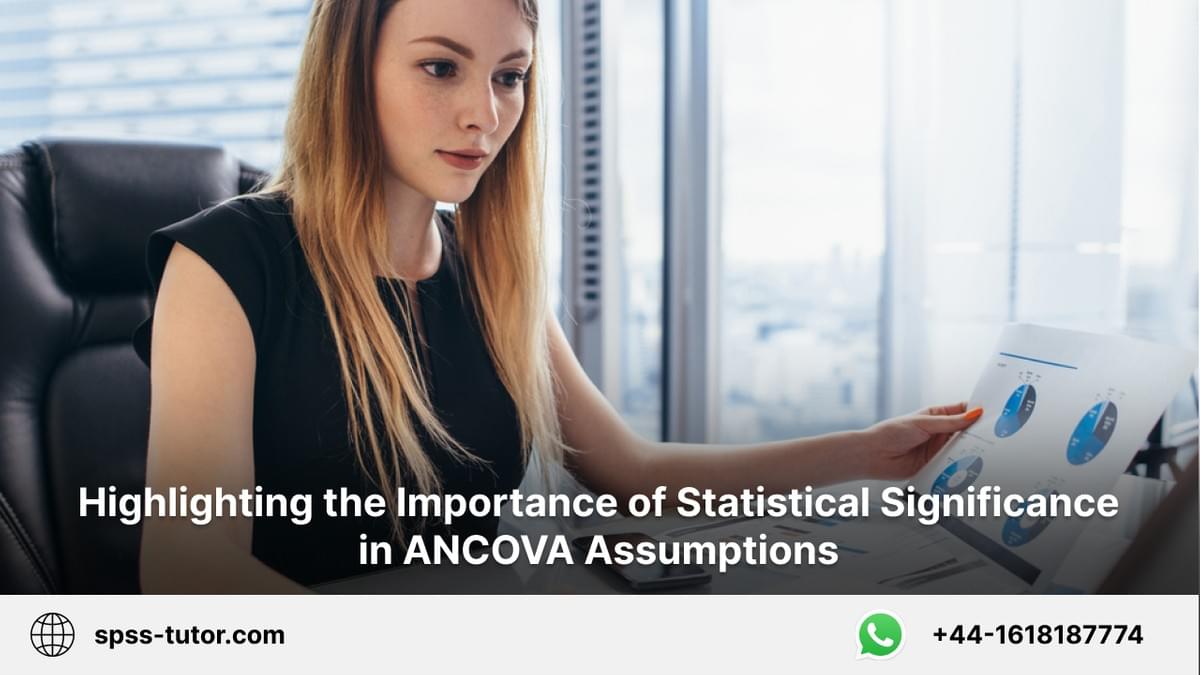
Introduction
ANCOVA is an analysis of covariance and SPSS help provides statistical assistance that is used to test the main and interaction effects of categorical variables on a continuous dependent variable, by controlling for the effects of the selected other continuous variables that co-vary with the dependent variables in the data set. The control variables are considered covariates. ANCOVA is mainly used for several purposes, including conducting experimental designs, controlling the factors which cannot be randomised but which can be measured on an interval scale as well as in observational designs, and removing the effects of the variables which modify the relationships of the categorical independents to the interval dependent variables.
Statistical significance in ANCOVA assumptions
ANCOVA is widely used by the researchers. In addition to this, in the regression models, in order to perform regressions, there are both categorical and interval independents and the ANCOVA is used. The key concepts are to identify the covariates, which is an integral level of independent variables. If there are no such covariates, the researchers can use ANOVA rather than ANCOVA. If there are covariates in the data set, ANCOVA is used widely. The F test and T-test are effective in conducting ANCOVA where the researchers try to review the values in the T-test and F test for drawing the final conclusion. F test of significance is used to test each main and interaction effects, for the case study analysis of a single interval dependent and multiple groups formed by categorical independent variables. The independent variables are more than two groups, where a normal sample population is considered. F is between the group's variance divided by within the group’s variances. If the computed p-value is small, then significant relations exist. Hence, significance is mandatory to be reviewed in order to implement the analysis of ANCOVA assumptions.
On the other hand, adjusted means are usually part of ANCOVA output and are mainly examined if the F test demonstrates the significant relationship that exists in the data set. Comparisons of the original and adjusted group means can also provide insight into the role of the covariates. For K groups are formed, by the categories of the categorical independent and measured on the basis of the dependent variables in the data set. In addition to this, the T-test is also effective for measuring the statistical significance in checking the assumptions of ANCOVA. A T-test of the significance of the differences in the means of the single interval is dependent, on the case of the two groups that are mainly formed by categorical independence. In this context, the ANCOVA can also be modelled by using regression, if the dummy variables are utilised for the categorical independents. When creating the dummy variables, the researchers or the data analysts try to use one less category than there are values of each independent. For full ANCOVA, one would also add the interactions for the cross predict terms equation, for each pair of the independent, including the equation of ANCOVA. Hence, one can also analyse regression by using ANCOVA in SPSS. The resulting F test will be the same as in the classical ANCOVA. F ration can also be computed by checking the assumptions and statistical significance, for accepting the alternative hypothesis in the research. Hence, the ANCOVA must ensure statistical significance, so that the researchers can gather relevant and valid data for further in-depth critical analysis to evaluate the impacts of the independent variables on the dependent variables in the data set.
As per the assumptions, the independent variables are categorical, except the least one that must be a covariate or interval level. Whereas, at least one independent must be categorical. The dependent variables are on the other hand continuous and interval levels. Hence, statistical significance must be checked to meet these two major assumptions for further statistical analysis by ANCOVA. The covariates variables are also continuous and interval level and these are assumed to be measured without an error. This ensures a low measurement error of the covariates. Covariates linearity or in known relationship to the dependent is also maintained for ensuring statistical significance in the data set so that it is effective for the researchers to progress in ANCOVA in a systematic process. The relationship between the dependent variables and the covariates must be known and most computer programs assumed this relationship is linear. The dependent man is mainly based on linear regression. In this context, the scatterplots of the covariates and the dependent for each of the k groups are formed by the independent data. Hence, statistical significance is playing a crucial role in maintaining data integrity and conducting ANCOVA for further critical analysis. In this context, multivariate normality is another major assumption in ANCOVA for the purpose of significance testing, in which the variables follow a multivariate normal distribution. It is one of the effective assumptions in ANCOVA in maintaining statistical significance.
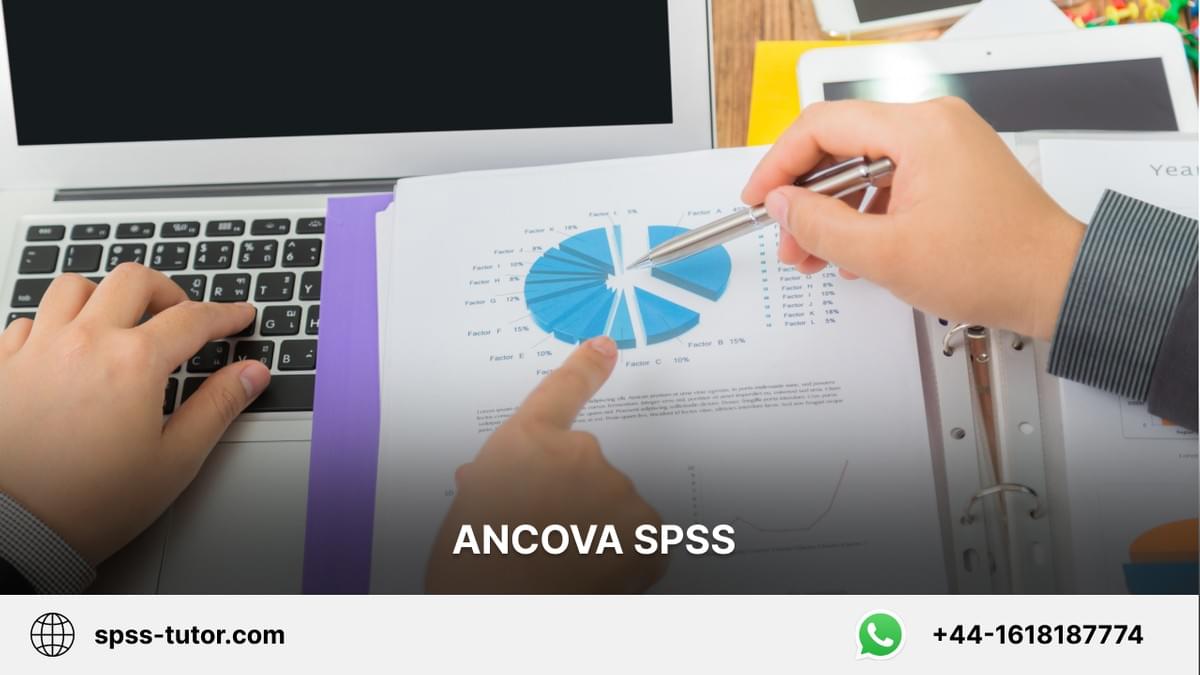
Conclusion
The other assumption in ANCOVA SPSS is the variance of the difference between the estimated means for any two groups is the same and compound symmetry states that the correlations between the two different groups are also the same value. Homogeneity of variance must be checked in the cells formed by the independent categorical variable. Independence of the error term is mandatory to be maintained for ensuring statistical significance and in this regard, the error term is independent of the covariates and the categorical independents, randomisation in the experimental designs assures this assumption will be met successfully. Homogeneity of the covariates regression coefficient is also important to ensure statistical significance for testing the research hypothesis. The covariate coefficient is the same for each group formed by the categorical variables and measured on the dependent variables. Hereby, the researchers and data analysts are concerned about maintaining the statistical significance of the data variables in order to meet the assumptions of the ANCOVA analysis so that they can perform efficiently and test the hypothesis critically.