Overall Understanding of Descriptive Statistics
Overall Understanding of Descriptive Statistics
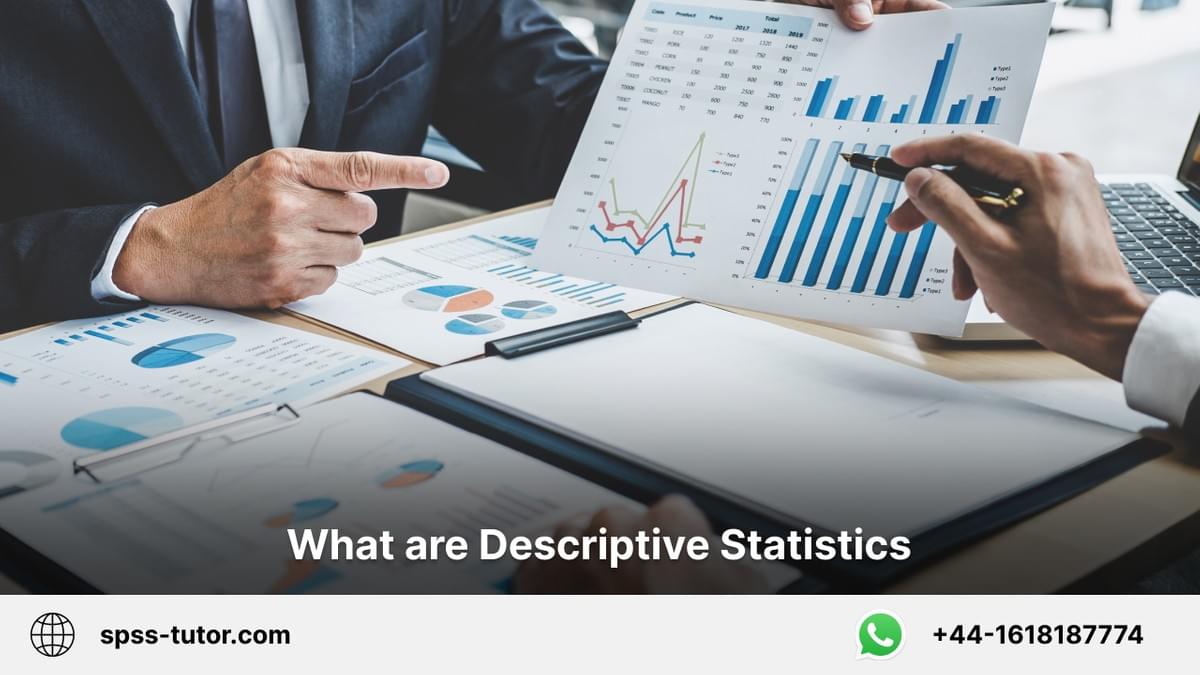
Introduction
Statistics, a captivating field encompassing the fusion of science and mathematics, delves into the intricacies of collecting, classifying, examining, understanding, and artfully presenting numerical facts and data. This is beneficial when dealing with populations too large and widespread for specific, detailed measurements. Statistics are necessary to conclude a dataset from a data sample.
Statistics is further divided into two types: descriptive and inferential. Descriptive statistics are mainly used in the methodology section of the thesis. Descriptive statistics summarise and meaningfully present this data to understand the underlying information quickly. Today, let's look at descriptive statistics, including definitions, types of descriptive statistics, and the difference between descriptive and inferential statistics.
What are Descriptive Statistics?
In the field of statistics, there exists a branch known as descriptive statistics, which undertakes the difficult task of summarising, organising, and presenting data with utmost importance and brevity. The essence of this discipline lies in carefully describing and analysing the key features and characteristics of datasets without making any bold assumptions or sweeping conclusions regarding the wider population.
The ultimate purpose of descriptive statistics is to present a clear and concise summary of the data, enabling researchers and analysts to gain deeper insight into the dataset's underlying patterns, trends, and distributions. This comprehensive summary usually includes measures of central tendency (e.g., mean, median, mode), dispersion (e.g., range, variance, standard deviation), and configuration of the distribution (e.g., skewness, kurtosis).
Beyond numerical summaries, descriptive statistics involves an artistic depiction of data through multiple charts, graphs, and tables, which enhance visual understanding and interpretation of the information at hand.
The standard graphical techniques employed are:
- Great histogram.
- Magnificent bar chart.
- Delectable Pie Chart.
- Whimsical scatter plot.
- Mysterious box plot.
By harnessing the power of descriptive statistics, researchers can efficiently encapsulate and clarify the quintessential features of a dataset, foster a better understanding of the data, and lay the foundation for further statistical analysis or informed decision-making processes.
- Purpose of descriptive statistics : Descriptive statistics aim to summarise and present data naturally and understandably. The significant features and patterns of the dataset are succinctly and plainly explained.
- descriptive data analysis : Aims to concisely and effectively present facts. It aids in decision-making, the identification of data trends, and the comparison of multiple datasets. The use of graphical representations improves data transmission and data verification. Descriptive statistics also aid in creating theories and research strategies for more involved investigations.
- Analysis of Variance in Descriptive Statistics : Descriptive statistics summarise information and depict data that reflect importance and depth. They offer a brief but informative glimpse into the essential characteristics of a dataset without going beyond the scope of the raw data or rendering any definite conclusions. Below, we've featured a host of generic descriptive statistics, each laced with a delectable mix of specific words to enhance their originality:
Measures of central tendency
- Mean: The arithmetic mean represents the total of all the data points.
- Median: The median of a dataset is located in the middle when the data is ascending or descending.
- Mode: The value that occurs most frequently among datasets.
Measures of Dispersion or Variability
- Range: The gap spanning from the highest maximum to the lowest minimum value within the dataset.
- Variance: The average magnitude of the squared difference calculated between each data point and the mean.
- Standard Deviation: The optimal representation of the mean deviation of the mean, unveiled as its square root.
Status Measures
- Percentile: A percentile subdivision divides the dataset into one hundred equal divisions, each containing the corresponding percentage of the data points below it.
- Quartile: The quartile partition separates the dataset into four equal parcels, each comprising 25% of the data.
- Interquartile Range (IQR): The gap between the most prominent quartile (Q1) and the third quartile (Q3).
Frequency Distribution
The tabulation and presentation of data in a tabular expression show examples of each value or range of values.
- Skewness : Analysing how skewed the data distribution is. Positive skewness reflects a rightward elongation of the tail, whereas negative skewness implies a leftward elongation.
- Kurtosis : Calculating the distribution of the data's form. Low kurtosis denotes a relatively level distribution, while high kurtosis reveals a distinct distribution.
- Cross-Tabulation (Contingency Tables) : Displaying the joint distribution of two categorical variables is a technique to explore the complicated relationships between them.
- Histogram : A frequency distribution is represented graphically, with each bar representing the number of occurrences within a given interval.
- Box Plot (Box-and-Whisker Plot) : A brief, graphical representation of the data distribution that deftly displays the median, quartiles, and any possible outliers. This artistic portrayal provides insight into the data's central tendency, variability, and dispersion for researchers and analysts looking to grasp the unique characteristics present in existing datasets.
Best Tools for Descriptive Statistics
SPSS (Statistical Package for the Social Sciences) is widely used for statistical analysis in various fields, particularly the social sciences. Among other statistical techniques, computing descriptive statistics makes extensive use of it. Researchers and analysts can effectively analyse and summarise data using SPSS help, getting essential insights into the dataset's properties. A vital tool for doing descriptive statistical analysis, it offers a user-friendly interface for producing measures of central tendency, variability, and graphical representation.
Descriptive statistics are often essential for the evaluation and interpretation of data. It transforms unstructured data into meaningful information that audiences can understand. The foundation for learning from data and drawing conclusions in business, research, or everyday decision-making is provided by descriptive statistics.
Difference Between Descriptive and Inferential Statistics
Descriptive statistics paraphrase and present data meaningfully and compactly, describing essential characteristics and patterns within the dataset. It focuses on measures of central tendency, variability, and graphical representation to acquire sapience. On the other hand, deducible statistics use sample data to make consequences or prognostications about larger occupants. It tests suppositions, determines connections between variables, and assesses the significance of findings. While descriptive statistics give a shot at the data, deducible statistics extend the analysis to draw broad conclusions and make prognostications beyond the observed sample.
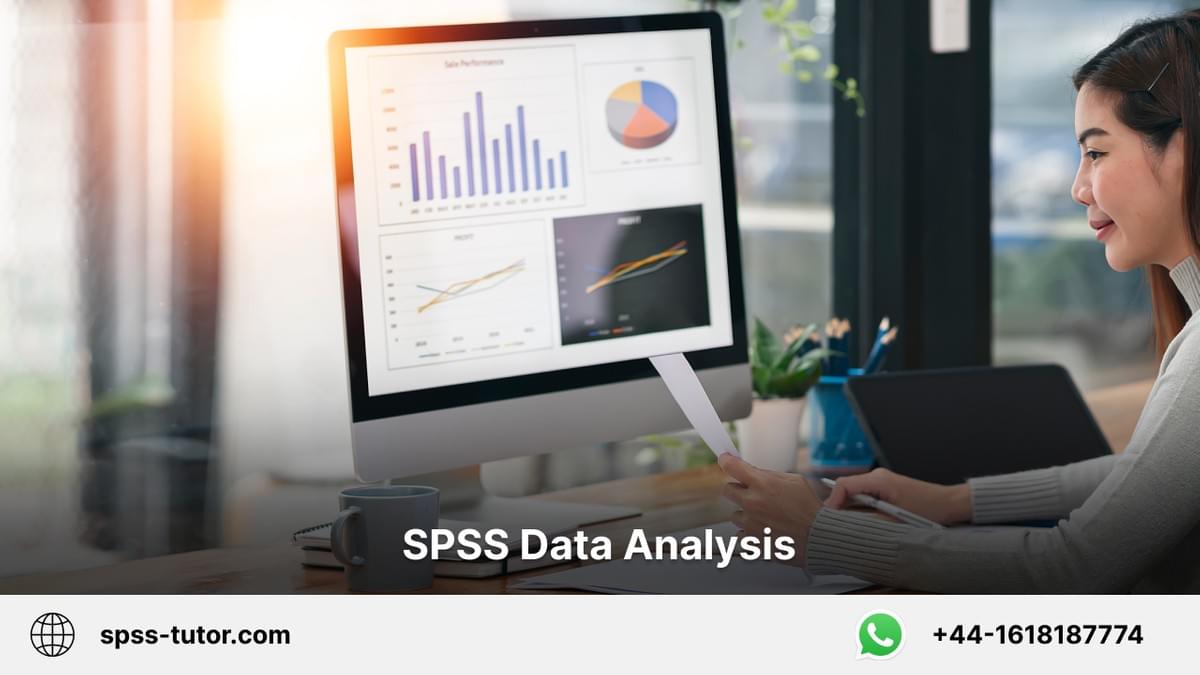
Conclusion
Descriptive statistics, combined with the critical data analysis capabilities of SPSS, grease a comprehensive understanding of datasets across eclectic disciplines. By using the tools of SPSS to their complete eventuality, experimenters and judges can gain precious perceptivity, make informed opinions, and contribute to progress in their separate fields. Combining descriptive statistics and SPSS data analysis opens new avenues for knowledge discovery and enhances the quality of exploration and decision-making. You can visit SPSS Consulting Services to learn further about SPSS Support. They give assignment help with SPSS data analysis.